arxiv上的一篇preprint文章,通过构建更复杂场景的数据集进行阴影检测。论文没有详细看,我关注的重点在特征融合模块,这里的特征融合还是具有不错的参考价值的。
low-level feature maps包含细节信息,可以帮助discover shadow boundaries and tiny shadows;而深层次的features contain global context information to help recognize the shadows,因此这里使用如下的结构进行特征融合,也就是detail enhance module。
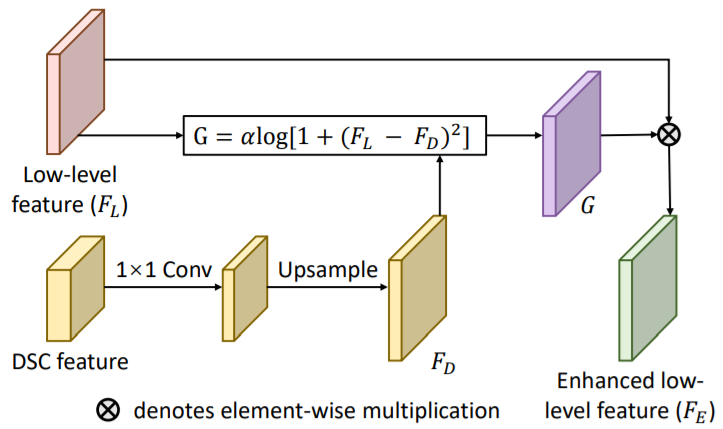
使用log函数衡量深层特征和浅层特征的距离,再引入alpha参数作为可学参数进行gate,没有引入过多参数量。
使用该模块可以enhance the spatial details and produce the refined low-level feature。
整个网络的Pipeline如图
下面的表格是ablation study,使用DEM后效果更好